- Interview
HBP scientist Svenja Caspers uses big data to better understand why brains vary so much in ageing
21 September 2022
Every brain is different, and the same structural change may cause loss of function in one brain, but have no consequences in another. In order to better understand why individual brains vary so much, scientists at the Human Brain Project (HBP) are using brain models and simulations.
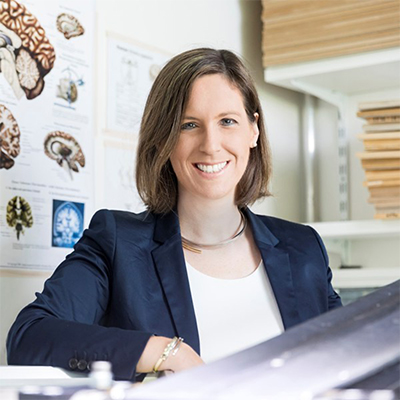
In this interview, Svenja Caspers talks about her research at the Human Brain Project (HBP), which explores the brain and its variability, especially during ageing.
Svenja Caspers is professor for Anatomy of the Institute for Anatomy I, Heinrich Heine University Düsseldorf, and leader of the "Connectivity" Working Group and Deputy Director at the Institute of Neuroscience and Medicine (INM-1) at the Forschungszentrum Jülich, in Germany.
You investigate the relations between brain structure, function and connectivity in the course of ageing. What is the main purpose of this research?
The major goal of this line of research is to understand why and how the brains of different individuals vary so much - particularly as we age - and what factors might be responsible for that.
There are genetic influences, which are known to cause – or at least partly cause – neurodegenerative disorders, and some of them could also influence the ageing brain itself, even in the absence of any particular disorder. There are also all the other influencing factors from our surroundings, from our environment, the way we live, our lifestyle: drinking alcohol, smoking, doing sports, how we eat and what we do every day. As we age, the effects of such factors accumulate.
We do see a lot of inter-individual variability, particularly in the ageing brain. The idea is to better characterise the normal range of ageing as compared to some extremes, like the diseases which might occur someday sooner or later.
What methods do you use?
The typical methods that we have been using are magnetic resonance imaging on large cohorts of thousands of subjects, to measure in vivo their brain structure, functional network architecture and connectivity between different brain areas, and having in addition to that blood tests, questionnaires and cognitive testing. These are then analysed using high-performance computing clusters.
How has the HBP facilitated this research?
Within the HBP, we are closely collaborating with colleagues, particularly with Viktor Jirsa and his group in Marseille. We include our knowledge, our acquired results on ageing as an example of how variability might work in the brain, into a brain model and simulation. We would like to understand what influences the brain and how it changes. If we assume certain changes in the brain and model these, we can compare the output of these data-driven models to our experimental data. This way we test whether our hypotheses of how the brain changes and reorganises are confirmed by the output of the models.
What are currently the greatest challenges in this field?
One of the biggest challenges is to bring all of the different individual factors that might influence us together into a useful framework. Each of the different factors might have little effects on the brain, but all of them together might have quite a heavy influence. As individuals, we are all affected by different factors, so it's basically a statistical problem. But there are a lot of endeavours going on beyond classical statistics, towards machine-learning or deep-learning approaches, which require large cohorts, to have enough training data. That's one major challenge in the population imaging field.
In the HBP, we have the benefit of being able to study people in vivo and see their behaviour, while we are doing population imaging. But we do not know enough about the smaller scales of brain organisation, the cellular or the molecular level. You can only see the brain as a whole in the imaging situation. The HBP’s effort to integrate cross-scales into the models is a great endeavour that allows us to refine the models. Hopefully, the results will resemble features of the living human brain more closely.
Which factor has a greater impact on the ageing process of the brain – genetics or lifestyle?
We have known for quite a while that lifestyle factors such as smoking and cardiovascular risk, non-beneficial diet, metabolic syndrome, diabetes and others have a strong impact on the brain and could cause an accelerated ageing process. At the same time, some genetic factors may be more relevant than others, for example, those influencing Alzheimer’s or Parkinson’s disease.
I’m hesitant to say that there is one major factor that might be the most relevant. All the individual factors contribute only slightly to what happens to our brain, but the combination of several of these factors and their mutual interactions is more relevant.
“The HBP effort to integrate cross-scales into brain models is a great endeavour that leads to better results”
Within the HBP, you are working on a showcase project on brain models. What is the goal of this work?
The showcases are conceptualized as examples for the usability of the capabilities of EBRAINS and the modelling behind it. Our showcase is an example to demonstrate how model building can help us understand the brain and its variability. We used age as the first factor to be implemented, because this is abundantly available. And age is a factor for which at least some theories exist on how it might affect the brain in a systematic way. There are general rearrangements in the brain that we thought could be best exemplified in a model-based approach, where we can formulate a hypothesis of what we would expect to see if the model works. Now that we have this working model, we would like to widen our view and integrate other factors as well.
How does this work help to address the issues of healthy ageing in the population?
This contributes to a better understanding of healthy ageing because eventually we can use such a brain network model to predict what happens in an individual subject's brain. If we have trained the model on several ideas and cohort data, then we could predict with a new individual brain how that particular subject would be affected by a given outside factor. Eventually, it could be helpful to better individualise and personalise treatment options.
That is the general idea of our effort in Showcase 1. We would like to tease the possibilities that we have now from the experimentalists and the modellers to get something that could help the clinicians. In the end, such models could benefit clinicians who one day could generate a virtual twin from the patients to see whether their subject brains would benefit from given inputs.
There’s still a long way to go, but this is the direction through which it goes.
The interview was conducted by Helen Mendes.