Brain-Inspired Cognitive Architectures
Contact us
Contact us via email at support@ebrains.eu
Community
Join the Brain-Inspired Adaptive Cognitive Architecture Space in the EBRAINS Community
From advanced learning to neurorobotics and neuromorphic applications
The central ambition of this focus area is to better understand how brain networks enable visuo-motor and cognitive functions, such as dexterous manipulation, spatial navigation, and relational reasoning.
Our approach has been to emulate the architecture and operation of the brain by designing functional cognitive architectures addressing challenging visuo-motor and cognitive problems. Numerical simulations and embodiment in virtual or real robots enabled us to study closed-loop interactions between the brain, the body, and the physical environment. We targeted the development of a novel cognitive framework supporting the flexible integration of a range of heterogeneous modules, representing brain areas, into coherent embodied architectures. Furthermore, we developed new learning rules to train the modules in biologically-plausible ways and new neuromorphic computation methods for rapid, energy-efficient simulation of large-scale models. Our computational architectures can perform a variety of cognitive functions and led to new experimentally-testable hypotheses about brain function.
Dexterous manipulation - how the brain coordinates hand movements
The ability to dexterously manipulate objects is essential to our everyday life. In Showcase 5, we shed light on the neural computations underlying this complex task in a goal-driven approach. To that end, we trained an artificial agent controlling a simulated anthropomorphic robotic hand with reinforcement learning to perform ecologically valid, visually-guided actions to manipulate an object. These actions were computed in a recurrent convolutional neural network modelling the primate brain’s frontoparietal network involved in visually-guided hand and arm movements. The neural computations that emerged during training can now be used to build new hypotheses about their biological counterpart.
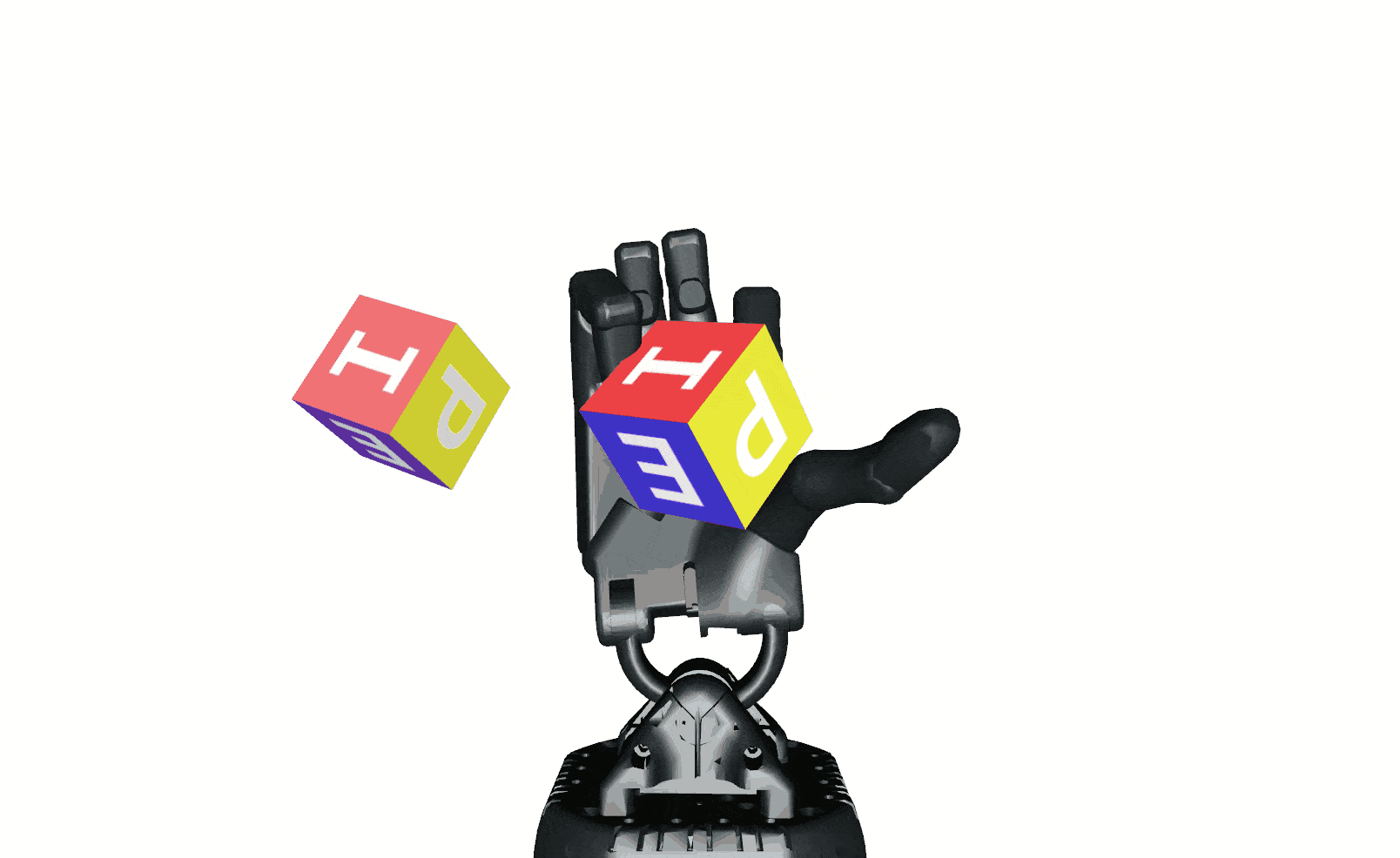
Brain-based technology to support safe human-robot collaboration
The central aim of Showcase 6 is to demonstrate the potential of functional cognitive models in cobotics applications. It addresses, among others, relevant research questions regarding safety for human beings in a shared robotic workspace. The showcase connects multiple models developed by HBP partners into a single simulated physical environment. Namely, these models comprise a visual system to detect humans and robots reliably in the demonstrator scene, a high level planning module allowing the robot to generate smooth and efficient trajectories as well as a musculoskeletal model accurately representing a human in the scene. By combining the capabilities of the individual models, we can control a robotic arm in a safe and efficient manner, allowing us to perform complex tasks in collaboration with a human. We demonstrate a range of operations, from simple tool handovers to dedicated collaborative part assembly, similar to what one might find in an automotive factory.
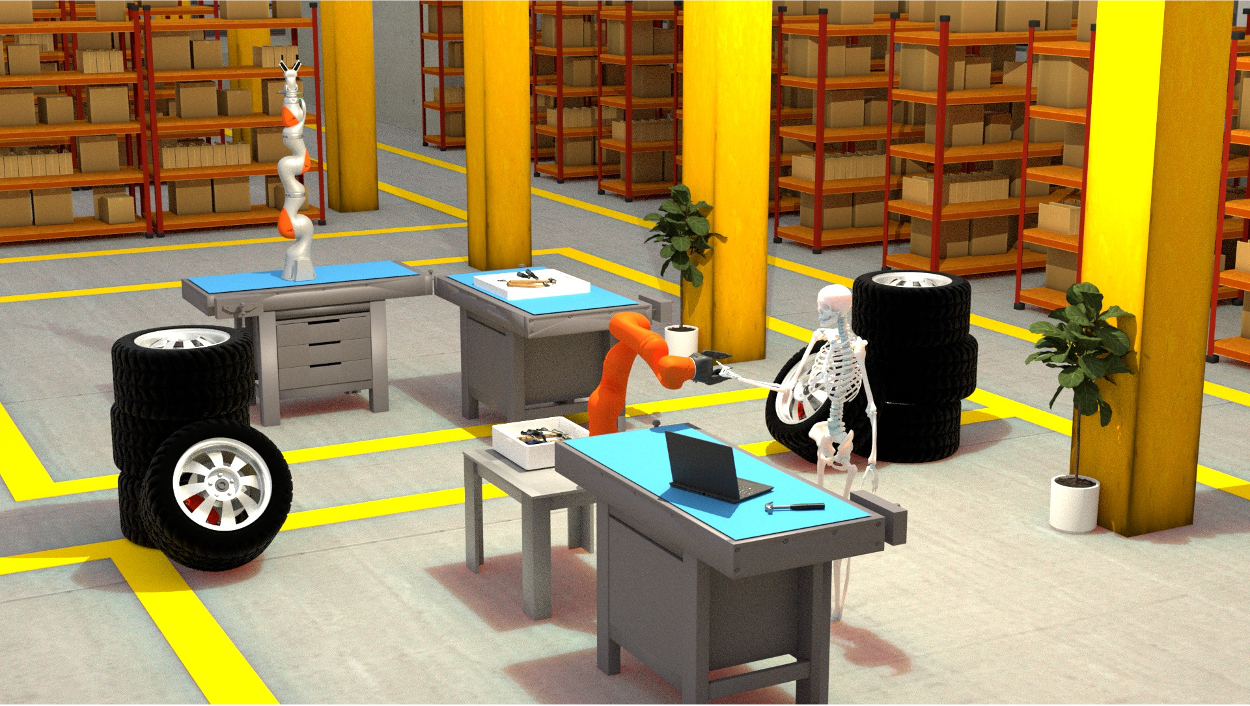
Subtopics
Brain-Inspired Artificial Intelligence
As opposed to current AI networks, the human brain has rich internal dynamics, is data- and energy-efficient, and can learn a large variety of tasks continuously with limited supervision by interacting with the (physical and social) environment. Furthermore, in contrast to a homogenous deep neural network, the human brain has a sophisticated network architecture consisting of many brain areas organised into networks and cortical systems that are recruited in context- and task-dependent ways, to fulfil a wide range of cognitive challenges. Thus, scientists working in this subtopic build biologically-inspired cognitive architectures that consist of many networks or modules that work together to create more complex and flexible behavior than individual deep networks (see Showcase 5 and 6). The modelling approach integrates architectural constraints from the brain but derives network parameters by using biological learning. As a result of modularity, a single module can often serve as a building block for many different architectures. The architectures are extended by adding increasingly more higher-level cognitive abilities, including neural systems for relational reasoning and hierarchical planning and by embodying them into robots able to address ecologically valid tasks, such as navigation and manipulation.
The BrainProp algorithm as outlined in Pozzi et al, 2020. Only one output unit, corresponding to the selected action or class, is trained at a time by means of an attentional feedback sign
Relevant Publications
People and Groups
Biophysical Modelling
This subtopic is concerned with the development of cellular-resolution computational models of the brain combining biological realism with learning and task performance. A particular aim was to gain insight into visuomotor processing through models of multiple brain areas including the cerebellum, basal ganglia, cerebral cortex, hippocampus, and other intercalated nuclei. Aspects taken into account encompassed spiking communication, cell-type-specific electrophysiology and connectivity, spike-timing-dependent plasticity, and dopamine-dependent reinforcement learning. These models formed a bottom-up complement to the top-down approach taken elsewhere within the focus area, and have suggested mechanisms for cognitive architectures more closely based on known anatomy and physiology. Besides the neuroscientific knowledge gained from these models, they constitute testbeds for various EBRAINS services: simulation, high-performance computing, neuromorphic computing, and neurorobotics.
Activation of a vertical column of the cerebellar cortex (as shown in Schepper et al, 2021)
Relevant Publications
Korcsak-Gorzo A, Müller MG, Baumbach A, Leng L, Breitwieser OJ, et al. (2022)"Cortical oscillations support sampling-based computations in spiking neural networks" PLOS Computational Biology 18(3): e1009753.
N. R. Luque, F. Naveros, I. Abadía, E. Ros, A. Arleo. (2022) “Electrical coupling regulated by GABAergic nucleo-olivary afferent fibres facilitates cerebellar sensory-motor adaptation” Neural Networks, doi: 10.1016/j.neunet.2022.08.020.
Geminiani A., Mockevičius A., D'Angelo E., Casellato C. (2022) "Cerebellum Involvement in Dystonia During Associative Motor Learning: Insights from a Data-Driven Spiking Network Model" Front Syst Neuroscience, doi: 10.3389/fnsys.2022.919761.
Fruzzetti L., Kalidindi HT., Antonietti A., Alessandro C., Geminiani A., Casellato C., Falotico E., D'Angelo E. (2022) "Dual STDP processes at Purkinje cells contribute to distinct improvements in accuracy and speed of saccadic eye movements" PLoS Comput Biology, doi: 10.1371/journal.pcbi.1010564.
Antonietti A., Geminiani A., Negri E., D'Angelo E., Casellato C., Pedrocchi A. (2022) "Brain-Inspired Spiking Neural Network Controller for a Neurorobotic Whisker System" Front Neurorobotics, doi: 10.3389/fnbot.2022.817948.
De Schepper R., Geminiani A., Masoli S. et al. (2022) "Model simulations unveil the structure-function-dynamics relationship of the cerebellar cortical microcircuit" Communications Biology 5, 1240, doi: 10.1038/s42003-022-04213-y.
Grillo M., Geminiani A., Alessandro C., D'Angelo E., Pedrocchi A., Casellato C. (2022) "Bayesian Integration in a Spiking Neural System for Sensorimotor Control" Neural Computation, doi: 10.1162/neco_a_01525.
González-Redondo Á., Garrido J., Naveros Arrabal F., Hellgren Kotaleski J., Grillner S., Ros E., (2023) "Reinforcement learning in a spiking neural model of striatum plasticity" Neurocomputing 548, 126377, doi: 10.1016/j.neucom.2023.126377.
van Albada SJ., Pronold J., van Meegen A., Diesmann M.(2021) "Usage and Scaling of an Open-Source Spiking Multi-Area Model of Monkey Cortex" Lecture Notes in Computer Science, doi: 10.1007/978-3-030-82427-3_4.
Tiddia G., Golosio B., Albers J., Senk J., Simula F., Pronold J., Fanti V., Pastorelli E., Paolucci PS., van Albada SJ. (2022) "Fast Simulation of a Multi-Area Spiking Network Model of Macaque Cortex on an MPI-GPU Cluster" Frontiers in Neuroinformatics, Vol. 16, doi: 10.3389/fninf.2022.883333.
People and Groups
Uncovering new learning rules
Intelligence is our ability to learn appropriate responses to new stimuli and situations. During learning, neurons in association cortices become tuned to relevant features and start to represent them with persistent activity during memory delays. Scientists from various groups in the HBP developed biologically plausible learning rules that explain how trial-and-error learning induces neuronal selectivity and working memory representations for task-relevant information. They examine the role of attention, synaptic tags, feedback connections and neuromodulators in learning, examining biologically plausible learning rules endowing neural networks to exhibit the same learning capacity and flexibility as the human brain. These projects addressed how neurons in the association cortex and subcortical structures transform sensory signals into activity patterns in the motor cortex that can guide optimal behaviour. Another hallmark of intelligence is to maintain and organise experiences in episodic memory, and to use these experiences for solving problems and for creating predictions of sensory inputs. Therefore, researchers worked on uncovering learning rules and spiking neural network architectures which can imagine possible consequences of taking a particular action, among other notable work. Intelligence is also characterised by the flexibility and adaptability of the responses to stimuli and situations. In line with this, biologically plausible learning rules and architectures were developed for solving multi-step visual tasks requiring the flexible composition of different subtasks, as well as learning-to-learn tasks requiring the learning of an overarching rule applied independently of the stimuli presented.
Biologically plausible implementation of natural-gradient-based plasticity for spiking neurons which renders learning invariant under dendritic transformations (Kreutzer, E., et al., 2022)
Relevant Publications
Mollard S, Wacongne C, Bohte SM, Roelfsema PR. (2023) "Recurrent neural networks that learn multi-step visual routines with reinforcement learning" bioRxiv. 2023.07.03.547198, doi: 10.1101/2023.07.03.547198.
van den Berg AR, Roelfsema PR, Bohte SM. (2023) "Biologically plausible gated recurrent neural networks for working memory and learning-to-learn" bioRxiv, 2023.07.06.547911. doi: 10.1101/2023.07.06.547911.
People and Groups
Neuromorphic Computing
Physical neural networks represent one of the most promising venues for realising massively parallel, energy-efficient information processing with imperfect and noisy components. Our research focused on two aspects of neuromorphic computing. On the one hand, project partners developed applications for neuromorphic devices, with demonstrated ability to push the boundaries of state of the art in terms of combined performance and efficiency. On the other hand, they actively pursued the development of novel neuromorphic hardware and software that incorporate important functional components of our biologically inspired models, including modified neuronal firing mechanisms, particular types of neuronal morphology and various aspects of synaptic dynamics.
The BrainScaleS chip with false-colour overlay of some of its components
Relevant Publications
People and Groups
Neuromorphic Sensing
Complementary to neuromorphic computing, scientists at the Royal Institute of Technology in Stockholm worked on event cameras that mimiced the working principle of the human eye allowing low-latency and low-power perception in challenging real-time real-world settings. This research combined such event cameras with neuromorphic computing systems to provide perception for low-power always-on sensing on wearable devices, seamless human-machine interaction, or enable autonomous robots to interact in a world made for humans. Furthermore, researchers at the University of Hertfordshire explored circuits and processes for sensing in the olfactory system and translated them into algorithms running on the HBP Neuromorphic Platform, combined with devices for electronic gas sensing. The aim was to enable gas-based navigation for robots and environmental monitoring at the edge.
The dynamic vision sensor setup allowing control of a robotic arm
Relevant Publications
Pedersen JE., Singhal R., Conradt J. (2023) " Translation and Scale Invariance for Event-Based Object tracking" Neuro-Inspired Computational Elements Conference, doi: 10.1145/3584954.3584996.
Bermudez JPR., Plana LA., Rowley A., Hessel M., Pedersen JE., Furber S., Conradt J. (2023) "A High-Throughput Low-Latency Interface Board for SpiNNaker-in-the-loop Real-Time Systems" International Conference on Neuromorphic Systems (ICONS), doi: 10.1145/3589737.3605969.
People and Groups
Cognitive Neurorobotics
Can we develop robots that have human-like perception, action and reasoning? This subtopic investigates the development of digital brains to control robots and artificial systems, and at the same time, it gives tools to validate current computational neuroscience theories. It focuses on areas, such as: perception and adaptive control (e.g., safe human-robot collaboration), robust local learning, and neurosymbolic planning. For instance, we investigate functional cognitive models for model predictive control with spiking neural networks based on predictive coding approaches, and we study forms of representation learning that incorporate inductive biases, such as objects, to allow abstract planning.