Human multiscale brain connectome
We focus on building, validating and operating the human multiscale brain connectome and its variability. The main ambition is to develop personalised, biologically detailed brain network models capable of generating signals commonly used in human brain imaging, for applications in neuroscience and medicine.
By linking a multiscale human brain atlas to computational modelling integrating in-vivo and and ex-vivo data in the same brain reference framework, we aim to better understand the fundamental mechanisms of how the brain generates its behaviour and overcoming the challenges posed by inter-subject variability and neurodegeneracy.
Our goal is to increase the capacity of the neuroscientific community to model multiscale neural activity of human brain networks by building a conceptual, organisational and computational framework fully embedded in EBRAINS. Our vision is the translation of digital twin frameworks into clinical applications.
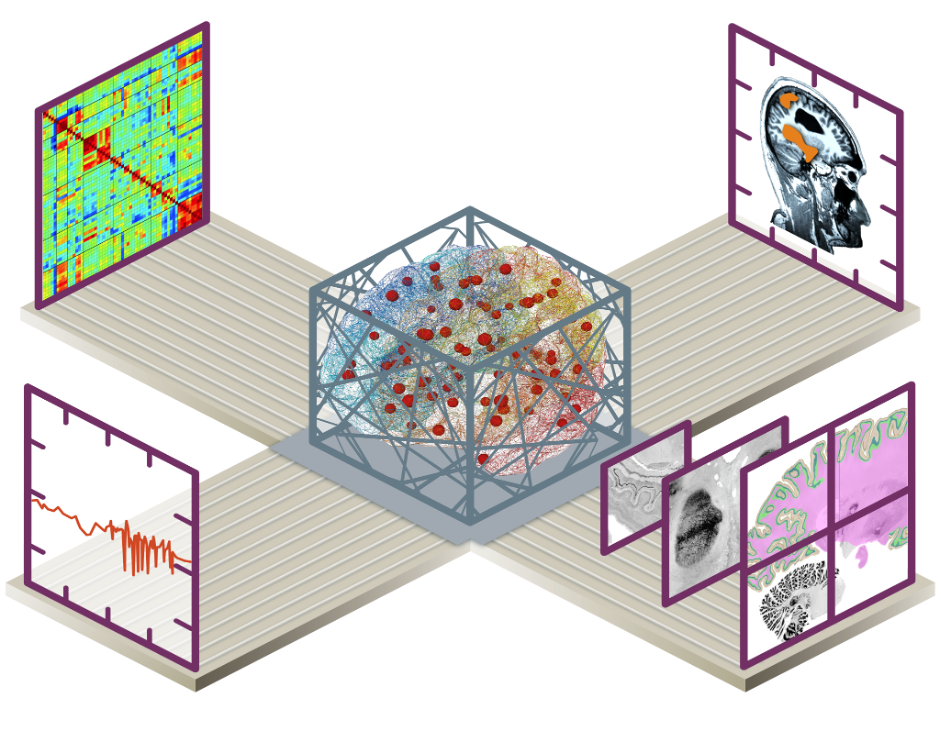
Introduction
Mapping the connections inside the brain, from the brain network down to the neuron scale, is fundamental for the understanding of how the brain works, how it can be modelled and simulated, and how to develop neurological applications, including clinical ones. Bridging the gap between highly detailed human brain atlases and computational modelling, we are able to better understand how the brain generates its behaviour, laying the groundwork for future theoretical and applied neuroscience. Capitalising on many years of HBP work, we assemble components to integrated workflows involving a battery of tools (bottom-up, top-down) interoperating data, atlases, multiscale models, and simulators. Several Showcases provide examples of such workflows integrated in EBRAINS. We focus on, but are not limited to, the human brain, and the creation of workflows for personalised brain models based on individual data. The validity of the principles and concepts is demonstrated in applications to individual patient data for clinical translation such as in epilepsy, and in cohort data to understand human variability such as in healthy aging and pathology. Neuroethical interrogations guide the debate on the role of the digital twin brain in society.
We have demonstrated that fusion of (typically ex-vivo) high-resolution data and (in-vivo) individual data improves personalised brain modelling, developed workflows for adapting deep learning techniques to brain model inversion, and progressed towards translation of personalised brain modelling technologies to clinics and adoption by industry partners, such in the case for epilepsy treatment.
We aim to establish a computational software interface between brain simulations and EBRAINS’ multilevel human brain atlas, which allows reproducible, structured and efficient use of parcellation maps and regional data features in simulations. A particular emphasis is to link information from in-vivo imaging, capturing variance in the population, with information from microscopic imaging, capturing cellular detail and variance between and inside brain regions. Our project exploits human brain data currently available on EBRAINS (intracranial stereo-electroencephalographic SEEG datasets) and aims at providing easily accessible workflows for the neuroscientific community interested in the analysis and modelling of cognitive brain networks.
We produce informative personalised brain models that integrate multiscale brain connectomes as structural brain atlases capturing relevant cytoarchitectural features. To achieve this, we work on making the brain models biophysically interpretable to offer deeper understanding of generative mechanisms and informative metrics of the brain’s resting state. Since a complex set of interactions across scales accounts for brain function and dysfunction, in our approach we carefully take into account degeneracy and diagnostics of our analytics, which is at the core of responsible approach in personalised medicine. We develop a Bayesian model inversion framework in which structural and functional variations, that would be construed as “noise” in most perspectives, are considered as natural elements of the underlying rules of brain function. The Bayesian model inversion uses a battery of functional metrics, instead of a single biomarker, as such structural variability is incorporated in causal hypotheses about brain mechanisms that then drive whole-brain modelling of explanatory power on the nature of functional variability within and across individuals.
Showcase 1: Degeneracy in neuroscience - when is Big Data big enough?
Showcase 1 addresses one of the oldest enigmas in neuroscience, the dichotomy of brain structure and function. Individual brains differ from each other while maintaining full functionality in a given range of “normal” status. The objective of this Showcase is to demonstrate that cutting edge datasets on structural variability can be used to formulate causal hypotheses about brain mechanisms and then drive whole-brain modelling to explain functional variability within and across individuals. Human brain aging is a well-suited paradigm for this task as inter-individual variability in brain structure, function and cognitive abilities in older subjects is very pronounced and is a key obstacle on the way to a better understanding of aging. In Showcase 1, we integrate detailed multiscale data (brain connectome, region-specific data) in virtual brain models, implement the hypothesised aging mechanisms, and simulate the functional resting-state brain imaging data of a large cohort of individual brains.
Several recent advances have occurred with the development of novel high resolution techniques for neural field simulation inside the Virtual Brain software, including general purpose parameter inference techniques. At EBRAINS, we aim to develop and share computational and neuroinformatics tools for the analysis and modelling of functional brain connectivity mediating cognitive functions. Our approach combines data-driven tools for the characterisation of the role of interactions between brain regions during cognitive processes with inference and validation methods for the construction of computational models that reflect such empirical information.
Showcase 2: Improving epilepsy surgery with the Virtual Big Brain.
Clinical applications of HBP research include, but are not limited to, the improvement of epilepsy through the Virtual Epileptic Patient (VEP), as exemplified by Showcase 2. We construct a high-resolution brain model for improved prediction of epileptic seizure propagation. Epilepsy was simulated in the VEP model, representing the brain as a network of neural masses connected by white matter fibres. We extend this modelling approach to neural fields, where neural activity can be represented continuously across the spatial domain of the cortical surface. This gives rise to new neural dynamics such as travelling waves, which have been observed in empirical data, but could not be modelled using neural masses. We also extend the surface representation into the hippocampus, with the goal of increasing the details of this area in the future. This surface representation of neural activity will enhance the forward model for simulation through stereo-electroencephalography. Our model is combined with high-resolution connectivity data coming from the Chenonceau project. Before, connections were estimated between regions of a brain atlas. In the high-resolution model, we avoid the step of using a brain atlas and estimate the connections between vertices of the cortical mesh allowing for demonstrating more detailed seizure propagation dynamics.
Read more about Showcase 2 here.
Important publications
Hashemi et al. The Bayesian Virtual Epileptic Patient: A probabilistic framework designed to infer the spatial map of epileptogenicity in a personalized large-scale brain model of epilepsy spread. NeuroImage, Vol. 217 - 2020-08-01
Amunts et al. Julich-Brain: A 3D probabilistic atlas of the human brain’s cytoarchitecture. Science: Vol. 369, Issue 6506, pp. 988-992 - 2020-08-21
Casali et al (2020). Cellular-resolution mapping uncovers spatial adaptive filtering at the cerebellum input stage. Nature Communications Biology - 2020-03-15
Palesi et al. The Importance of Cerebellar Connectivity on Simulated Brain Dynamics. Frontiers in Cellular Neuroscience, Vol. 14 2020-07-31
Saggio et al. A taxonomy of seizure dynamotypes. eLife, Vol. 9 2020-07-21
Courtiol et al. Dynamical Mechanisms of Interictal Resting-State Functional Connectivity in Epilepsy. The Journal of Neuroscience, Vol. 40, No. 29 2020-07-15
Deco et al. Revisiting the global workspace orchestrating the hierarchical organization of the human brain. Nat Hum Behav. 2021 Jan 4.
Manninen T et al. Astrocyte-mediated spike-timing-dependent long-term depression modulates synaptic properties in the developing cortex. PLoS Comput Biol. 2020 Nov 10;16(11):e1008360.