Quick start
The neuromorphic compute services SpiNNaker an BrainScaleS can be accessed via the EBRAINS Research Infrastructure at https://ebrains.eu/nmc
Details: Please follow the "getting access" description to get started using SpiNNaker and/or BrainScaleS with an example collab and test quota on the EBRAINS research infrastructure (created by the HBP). Test access requires an EBRAINS account and is free of charge).
These videos of hands-on sessions and talks about the systems provide introductory to in-depth information.
Introduction
Neuromorphic computing implements aspects of biological neural networks as analogue or digital copies on electronic circuits. The goal of this approach is twofold: Offering a tool for neuroscience to understand the dynamic processes of learning and development in the brain and applying brain inspiration to generic cognitive computing. Key advantages of neuromorphic computing compared to traditional approaches are energy efficiency, execution speed, robustness against local failures and the ability to learn.
Neuromorphic Computing in the HBP and on the EBRAINS Research Infrastructure
In the HBP and the EBRAINS Research Infrastructure the unique neuromorphic systems are offered and further developed for external use.
The large-scale neuromorphic machines are based on two complementary principles. The many-core SpiNNaker machine located in Manchester (UK) connects 1 million ARM processors with a packet-based network optimised for the exchange of neural action potentials (spikes) (a comprehensive description is available in a free, open access book about SpiNNaker).
The BrainScaleS physical model machine located in Heidelberg (Germany) implements analogue electronic models of neurons and synapses.
Both systems are integrated into the EBRAINS Collaboratory and offer full software support for their configuration, operation and data analysis.
A prominent feature of the neuromorphic machines is their execution speed. The SpiNNaker system runs at real time, BrainScaleS is implemented as an accelerated system and emulates neurons at 1,000 times real time. Simulations at conventional supercomputers typically run factors of 1,000 slower than biology and cannot access the vastly different timescales involved in learning and development ranging from milliseconds to years.
Recent research in neuroscience and computing has indicated that learning and development are a key aspect for neuroscience and real-world applications of cognitive computing. The HBP was the only project addressing this need with dedicated novel hardware architectures.
Available systems
The BrainScaleS-2 single chip system with 512 point neurons or a lower number combined to structured neurons and with programmable plasticity is accessible for usage via PyNN, both for batch submissions and for interactive use via the EBRAINS Collaboratory. The system runs 1,000x faster than biological real time.
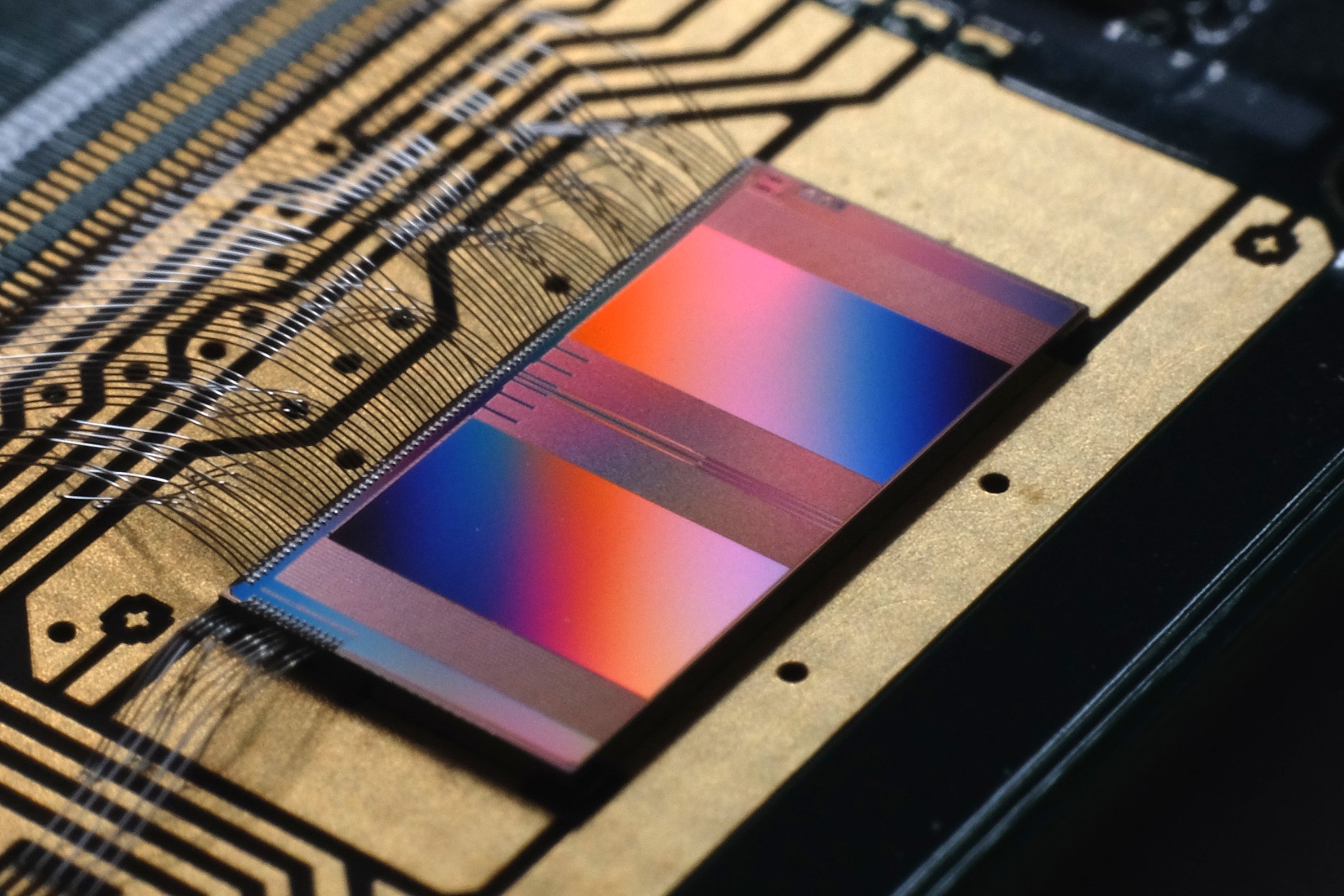
The (legacy) BrainScaleS-1 waferscale system is based on physical (analogue or mixed-signal) emulations of neuron, synapse and plasticity models with digital connectivity, running up to ten thousand times faster than real time.
The SpiNNaker system is based on numerical models running in real time on custom digital multicore chips using the ARM architecture. The SpiNNaker system (NM-MC-1) provides almost custom digital chips, each with eighteen cores and a shared local 128 Mbyte RAM, giving a total of over 1,000,000 cores.
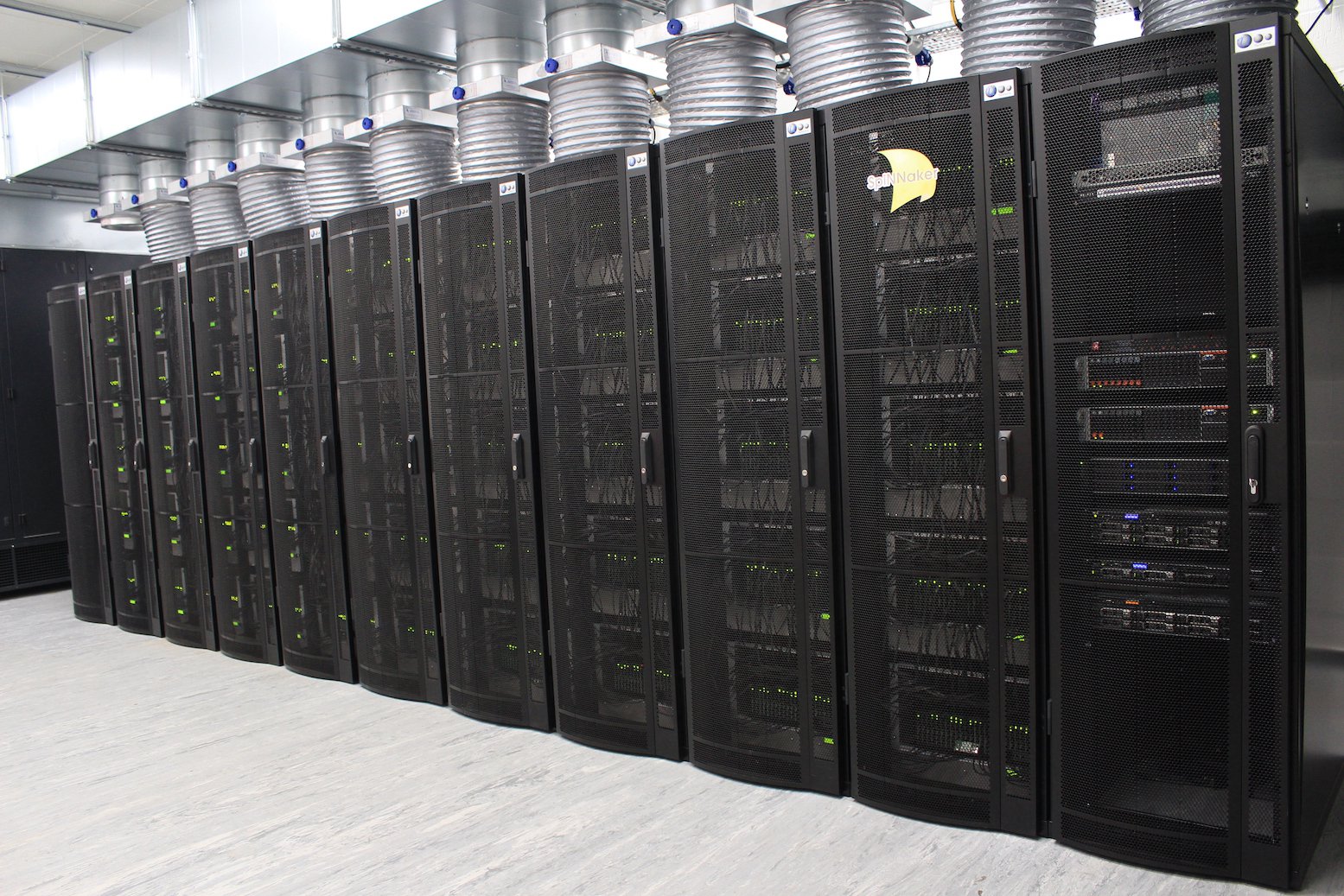
Outlook
A number of demonstrations of the benefits of neuromorphic technology are beginning to emerge, and more can be expected in the short to medium term. Various start-up companies are emerging, in the USA and elsewhere, to exploit the prospective advantages of neuromorphic and similar technologies in these new machine-learning application domains. In the HBP, small and large-scale demonstration systems are available and attract an increasing number of users from industry and academia. While these systems are primarily made for basic research on understanding information processing in the (human) brain, efforts are being made to also implement machine learning tasks on them. Next generation small-scale test chips of the SpiNNaker and BrainScaleS architecture are available for first test users since early 2018.
In the medium term we may expect neuromorphic technologies to deliver a range of applications more efficiently than conventional computers, for example to deliver speech and image recognition capabilities in smart phones. (Currently such capabilities are available only using powerful cloud resources to implement the recognition algorithms.) These will require small-scale neuromorphic accelerators integrated with the application processor, using a fraction of the resources of a single chip. Large-scale systems may be used to find causal relations in complex data from science, finance, business and government. Based on the causal relations detected, such neuromorphic systems may be able to make temporal predictions on different timescales.
In the long term there is the prospect of using neuromorphic technology to integrate energy-efficient intelligent cognitive functions into a wide range of consumer and business products, from driverless cars to domestic robots. While human-level “strong” artificial intelligence remains a mystery, and indeed may depend on the emergence of an understanding of information processing in the biological brain (through initiatives such as the Human Brain Project) before it becomes a practical reality, there are many useful applications that can benefit from more modest cognitive capabilities. The technology is relatively young, and there is much uncertainty as to where it will find its place in the wider world, but it clearly meets a need in the rapidly changing world of computing. The fact that major companies like IBM have defined cognitive computing as their main business for the future makes the development of neuromorphic hardware architectures especially interesting and economically attractive.
Target audience
The Neuromorphic Computing Platform targets researchers in multiple fields, including computational neuroscience and machine learning. Platform users are able to study network implementations of their choice including simplified versions of brain models developed on the HBP Brain Simulation Platform or generic circuit models based on theoretical work. The platform also offers industry researchers and technology developers the possibility to experiment with and test applications based on state-of-the-art neuromorphic devices and systems. Compared to traditional HPC resources, the Neuromorphic systems potentially offer higher speed (real-time or accelerated) and lower energy consumption. The accelerated systems are particularly suited for investigations of plasticity and learning, enabling simulation of hours or days of biological time in only a few seconds or minutes.
To request an account and then access the systems via the internet (free of charge for test usage) on the EBRAINS Research Infrastructure, please continue here.
What to expect?
The systems still have rough edges, but the platform offers user support and training, and the software supporting the platform is continuously being improved. Both systems (BrainScaleS and SpiNNaker) have an interface designed for neuroscience researchers, based on Python scripts using the PyNN API for simulator-independent specification of neuronal network models. PyNN scripts also run on the popular software simulators NEST, NEURON and Brian.
Contact
For any inquiries, please contact us via email or open a support ticket on EBRAINS.eu.
Community
During the Human Brain Project (which ended in September 2023) we also started offering a Neuromorphic Computing sub-community with event announcements in the EBRAINS community space. .
Publications
Project publications about neuromorphic computing.
208 Publications
Eric Müller, Arne Emmel, Björn Kindler, Christian Mauch, Elias Arnold, Jakob Kaiser, Jan V. Straub, Johannes Weis, Joscha Ilmberger, Julian Göltz, Luca Blessing, Milena Czierlinski, Moritz Althaus, Philipp Spilger, Raphael Stock,Sebastian Billaudelle, Tobias Thommes, Yannik Stradmann, Christian Pehle, Mihai A. Petrovici, Sebastian Schmitt, Johanne…
zenodo 2023-09-25Julian Göltz, Sebastian Billaudelle, Laura Kriener, Luca Blessing, Christian Pehle, Eric Müller, Johannes Schemmel, Mihai A. Petrovici
arXiv 2023-08-29Benjamin Cramer, Markus Kreft, Sebastian Billaudelle, Vitali Karasenko, Aron Leibfried, Eric Müller, Philipp Spilger, Johannes Weis, Johannes Schemmel, Miguel A. Muñoz, Viola Priesemann, Johannes Zierenberg
Physical Review Research, Vol. 5, No. 3 2023-07-19Jan Valentin Straub
- 2023-07-07Philipp Dauer, Milena Czierlinski, Sebastian Billaudelle, Andreas Grübl, Johannes Schemmel
2023 18th Conference on Ph.D Research in Microelectronics and Electronics (PRIME) 2023-06-18Elias Arnold, Georg Böcherer, Florian Strasser, Eric Müller, Philipp Spilger, Sebastian Billaudelle, Johannes Weis, Johannes Schemmel, Stefano Calabrò, Maxim Kuschnerov
Journal of Lightwave Technology, Vol. 41, No. 11 2023-06-01Philipp Spilger, Elias Arnold, Luca Blessing, Christian Mauch, Christian Pehle, Eric Müller, Johannes Schemmel
Neuro-Inspired Computational Elements Conference 2023-04-12Yannik Stradmann, Johannes Schemmel
Article in "7th HBP Student Conference on Interdisciplinary Brain Research" 2023-03-31Luca Blessing
2023-03-30Jakob Kaiser, Raphael Stock, Eric Müller, Johannes Schemmel, Sebastian Schmitt
arXiv 2023-03-28