- Research News
How methods developed for brain research can predict COVID spread
27 October 2022
Researchers of the Human Brain Project have applied methods and technologies that have originally been developed for the study of complex brain dynamics to study the spread of COVID-19, accurately predicting the timepoints at which new waves of infections started and the timepoints of their peaks and valleys. This epidemic model, one of the first to introduce psychological and socioeconomic factors into the equation, could be used to provide predictions for the spread of COVID-19 and other pandemic diseases in the future.
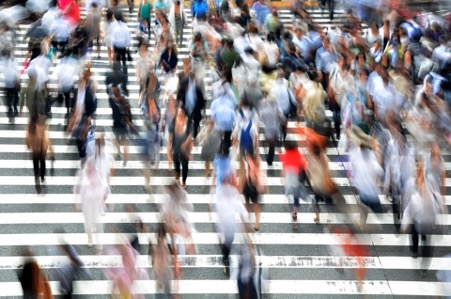
Despite becoming a part of our lives, the COVID pandemic still isn’t over, and with many nations preparing to strengthen their measures for the change of season, an informed approach to disease spread is still very important to reduce contagion without overbearing the population with restrictions. The new study by HBP researchers suggests that factors such as socioeconomic status, mobility, self-reported well-being and “lockdown fatigue” play a major role in determining the spread of the disease and should be taken into account to provide reliable predictions.
The study was carried out by the Institut de Neurosciences des Systèmes in Marseille, France, led by Viktor Jirsa, by a group which specializes in complex brain dynamics, mainly in the context of epilepsy. “We used the same class of algorithms for model inversion that we use in our personalized Virtual Epileptic Patient models,” explains Spase Petkoski, one of the authors of the study. “Epilepsy is a problem of a dynamic system with processes interacting at different timescales, much like the spread of a disease. In a sense, the spread of Covid-19 is an easier problem for us to solve, at least compared to the complexity of the human brain.” The researchers used data recorded in Germany and Denmark in the first month of the COVID pandemic, up to April 2020, and predicted the evolution for the months to follow (first results were published early on Medrxiv). While limited to those two countries, the researcher’s prediction matched the actual contagion curves registered in the following months.
Standard mathematical models of infectious diseases typically compartmentalize populations using the SIR labels (Susceptible, Infectious, Recovered), tracking people between groups as the spread progresses. “Our model further compartmentalizes the groups, dividing them into vulnerable and resilient, based on their socioeconomic status, self-reported well-being, how much they move and interact with others due to their work, how much time they have already spent under COVID restriction measures,” says Petkoski. For example, take an office worker with a decent pay and the ability of working remotely, and an essential worker who has to take public transport to go to work. A standard model might simply count them both as susceptible, but their economic condition, working arrangements, how much they are affected by restrictions and how much they move around can make a lot of difference in how they can catch and spread COVID. “According to our model, the effect of psychosocial causes on disease spread is almost equally impactful as governmental interventions and seasonality. This means that fighting socioeconomic consequences of COVID-19 is also a means of fighting its spread through non-pharmaceutical actions containing the disease,” adds Petkoski. “Our model also allows us to simulate what happens after the introduction of new measures, tracking how these events influence the contagion curves at multiple time scales.”
In addition to socioeconomic factors, well-being and mobility as reliable predictors, the study also suggests that without regular easing of restrictive measures, fatigue sets in: people are less likely to respect the measures and the risk of contagion probability increases. “Despite originating from brain research, models like the one we developed could be applied to COVID,” suggests Petkoski.
The results of the study were published in PLOS Digital Health.
Text by Roberto Inchingolo
Reference: Integrating psychosocial variables and societal diversity in epidemic models for predicting COVID-19 transmission dynamics, Viktor K. Jirsa, Spase Petkoski, Huifang Wang, Marmaduke Woodman, Jan Fousek, Cornelia Betsch, Lisa Felgendreff, Robert Bohm, Lau Lilleholt, Ingo Zettler, Sarah Faber, Kelly Shen, Anthony Randal Mcintosh, PLOS Digital Health, https://doi.org/10.1371/journal.pdig.0000098