- Press Release
Energy Efficiency of Neuromorphic Hardware Practically Proven
24 May 2022
Human Brain Project researchers collaborate with Intel to bring AI closer to the energy efficiency of the brain
Neuromorphic technology is more energy efficient for large deep learning networks than other comparable AI systems. This is shown by experiments conducted in a collaboration between researchers working in the Human Brain Project (HBP) at TU Graz and Intel, using a new Intel chip that uses neurons similar to those in the brain.
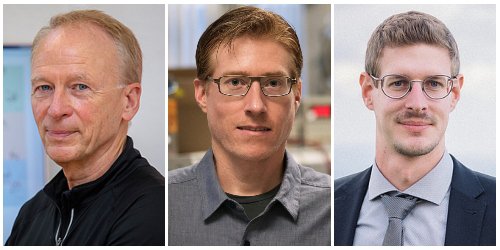
Smart machines and intelligent computers that can autonomously recognize and infer objects and relationships between different objects are the subject of worldwide artificial intelligence (AI) research. Energy consumption is a major obstacle on the path to a broader application of such AI methods. It is hoped that neuromorphic technology will provide a push in the right direction. Neuromorphic technology is modelled on the human brain, which is the world champion in energy efficiency. To process information, its hundred billion neurons consume only about 20 watts, not much more energy than an average energy-saving light bulb.
A research team from HBP partner TU Graz and Intel has now demonstrated experimentally for the first time that a large neuronal network on neuromorphic hardware consumes considerably less energy than non-neuromorphic hardware. The results have been published in Nature Machine Intelligence. The group focused on algorithms that work with temporal processes. For example, the system had to answer questions about a previously told story and grasp the relationships between objects or people from the context. The hardware tested consisted of 32 Loihi chips (note: Loihi is the name of Intel’s neuronal research chip). “Our system is two to three times more economical here than other AI models,” says Philipp Plank, a doctoral student at TU Graz’s Institute of Theoretical Computer Science and an employee at Intel.
Plank holds out the prospect of further efficiency gains, as the introduction of the new Loihi generation will improve energy-intensive chip-to-chip communication. This is because the neuronal communication within just one chip was even more outstanding. Measurements showed that the energy consumption here was even 1000 times more efficient, since no action potentials (the so-called spikes) had to be sent back and forth between the chips.
Mimicking human short-term memory
In their concept, the group reproduced a presumed method of the human brain, as Wolfgang Maass, Philipp Plank’s doctoral supervisor and professor emeritus at the Institute of Theoretical Computer Science, explains: “Experimental studies have shown that the human brain can store information for a short period of time even without neuronal activity, namely in so-called ‘internal variables’ of neurons. Simulations suggest that a fatigue mechanism of a subset of neurons is essential for this short-term memory.” Direct proof is lacking because these internal variables cannot yet be measured, but it does mean that the network only needs to test which neurons are currently fatigued in order to reconstruct what information it has previously processed. In other words, previous information is stored in the non-activity of neurons, and non-activity consumes the least energy.
Symbiosis of recurrent and feed-forward network
The researchers link two types of deep learning networks for this purpose. Feedback neuronal networks are responsible for “short-term memory”. Many such so-called recurrent modules filter out possible relevant information from the input signal and store it. A feed-forward network then determines which of the relationships found are really important for solving the task at hand. Meaningless relationships are screened out, the neurons only fire in those modules where relevant information has been found. This process ultimately leads to energy savings.
An important step towards energy-efficient AI
Steve Furber, leader of the HBP neuromorphic computing division and a professor of Computer Engineering at the University of Manchester, comments on the work by the Graz team:
“This advance brings the promise of energy-efficient event-based AI on neuromorphic platforms an important step closer to fruition. The new mechanism is well-suited to neuromorphic computing systems such as the Intel Loihi and SpiNNaker that are able to support multi-compartment neuron models”, said Furber, who is the creator of the SpiNNaker neuromorphic computing system, available on the HBP's EBRAINS Research Infrastructure.
TU Graz in the Human Brain Project
Within the Human Brain Project, the group of Wolfgang Maass is a key contributor to the research area “Adaptive networks for cognitive architectures: from advanced learning to neurorobotics and neuromorphic applications”. Here, the scientists work on brain-inspired algorithms for solving AI tasks and deep learning schemes for spike-based applications. The group takes inspiration from the way neurons communicate in the brain to investigate new methods for highly energy-efficient artificial neuron networks.
Media Contact:
Peter Zekert
Tel.: +49 2461 61 96860
press@humanbrainproject.eu
Funding statement
This research was financially supported by Intel and the European Human Brain Project, which connects neuroscience, medicine, and brain-inspired technologies in the EU. For this purpose, the project is creating a permanent digital research infrastructure, EBRAINS. This research work is anchored in the Fields of Expertise "Human and Biotechnology" and "Information, Communication & Computing", two of the five Fields of Expertise of TU Graz.
ABOUT THE HUMAN BRAIN PROJECT
The Human Brain Project (HBP) is the largest brain science project in Europe and stands among the biggest research projects ever funded by the European Union. It is one of the three FET Flagship Projects of the EU. At the interface of neuroscience and information technology, the HBP investigates the brain and its diseases with the help of highly advanced methods from computing, neuroinformatics and artificial intelligence, and drives innovation in fields like brain-inspired computing and neurorobotics.
New HBP brochure: Spotlights on latest scientific advances
Find the digital version here
ABOUT EBRAINS
EBRAINS is a new digital research infrastructure, created by the EU-funded Human Brain Project, to foster brain-related research and to help translate the latest scientific discoveries into innovation in medicine and industry, for the benefit of patients and society.
It draws on cutting-edge neuroscience and offers an extensive range of brain data sets, a multilevel brain atlas, modelling and simulation tools, easy access to high-performance computing resources and to robotics and neuromorphic platforms.
All academic researchers have open access to EBRAINS’ state-of-the art services. Industry researchers are also very welcome to use the platform under specific agreements. For more information about EBRAINS, please contact us at info@ebrains.eu or visit www.ebrains.eu.
IMAGES
The close-up shows an Intel Nahuku board, each of which contains eight to 32 Intel Loihi neuromorphic research chips. © Tim Herman/Intel Corporation
TU Graz scientist Wolfgang Maass deals with the question of how the information processing in the human brain works and how this could be used for computer applications. © Lunghammer - TU Graz